Ten Hurdles Slowing the Transformation
Artificial intelligence: How managers can clear the path faster for innovations.
05/2023
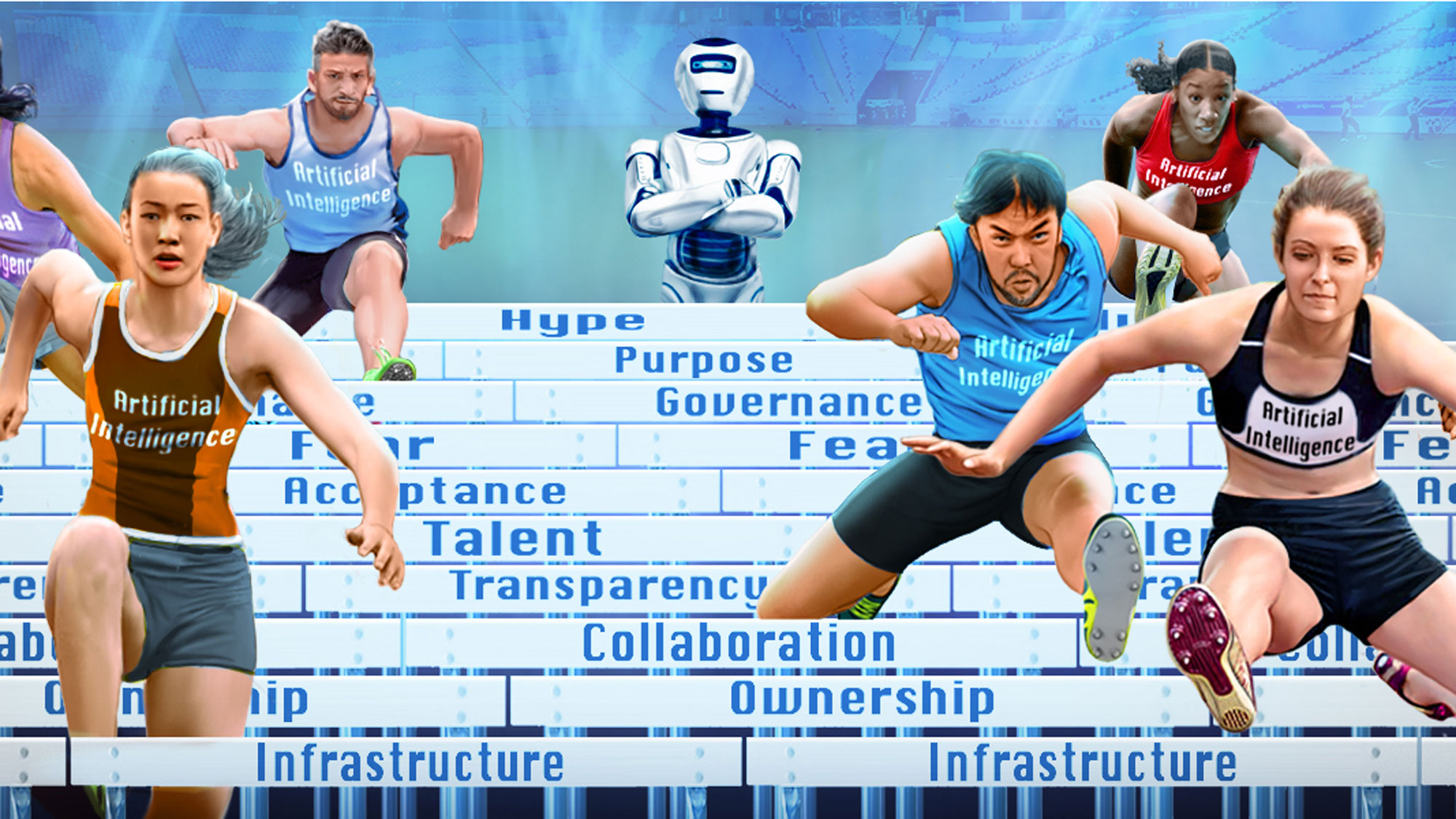
Machines that can learn, analyses of complex information with the help of big data, and key collaboration with digital assistants—companies and organizations are grappling with these innovations in the midst of a transformation. Hardly any sector can get away without doing so. In the future, tasks that are hazardous, highly labor or time-intensive, or burdened by huge volumes of data will be handled by automated systems supplied with artificial intelligence (AI).
Marching to the beat of digitalization, there is no alternative to this dynamic transformation. Managers seeking to create value to meet their competitive needs will have to anchor artificial intelligence in their value-creation processes and develop it further on a continuous basis. This is the only future path that can significantly increase efficiency and productivity. It is also the only way to ensure overall quality satisfaction on the part of customers—especially in highly competitive markets.
International experts consider the introduction of artificial intelligence to be as ground-breaking as the introduction of electricity, which was first put to practical use around 1600. Today, however, everything moves at a much faster pace—including the rate of progress. The online marketing industry is already advanced in its use of artificial intelligence, especially for analytics and optimization. Various other branches, by contrast, lag far behind. Why is that so? Formidable obstacles clearly stand in the way of digitalization—some within the companies themselves, but others having to do with the market or regulatory constraints.
An interdisciplinary team from the Porsche Consulting management consultancy composed of AI experts with a wide range of industrial experience has examined cases in practice. They have thoroughly analyzed the most common hurdles and formulated realistic approaches to overcoming them. The team has been applying these methods successfully with both domestic and international clients.
Direct contact to Porsche Consulting, department Artificial Intelligence and Data Analytics: ai@porsche-consulting.com