Artificial Intelligence:
5 Trends for 2025
From experiments to experience: Many companies have a clear artificial intelligence agenda for 2025 and beyond. The priority is on the most promising applications. And the most suitable methods. Porsche Consulting identified key trends — and presents five of them here.
12/2024
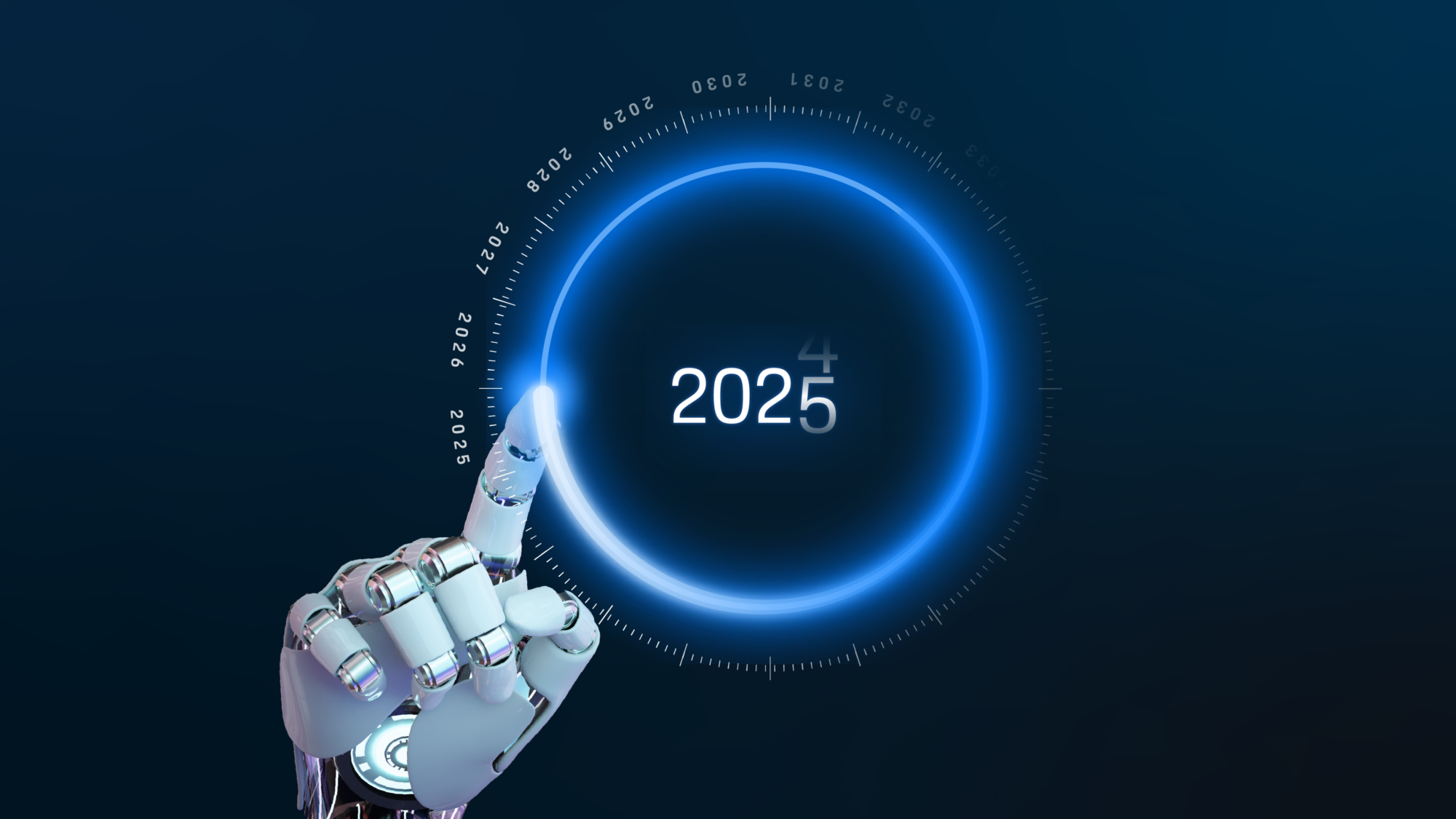
The time for experimentation has passed. The focus must now be on targeted utilization and concrete benefits. This, in a nutshell, describes Porsche Consulting’s hands-on experience with the new technology in a wide array of projects for clients from different sectors. Over recent years, companies have been launching many pilot projects for individual uses of artificial intelligence (AI). The main priority now, however, will be to rigorously evaluate further investments on the basis of their potential contribution to business success.
That means companies need to select the methods best suited to their needs from the comprehensive AI toolbox. And they have to make the transition from generic tools to customized solutions. Technical considerations are key to achieving better results, but companies also need to adapt their organizations in order to make full use of AI potential.
What tasks now head the agenda? And what paths lead to solutions? Drawing on its experience in practice, Porsche Consulting presents the following top trends:
Trend 1 The Method Matters
Situation: Attention is currently focused on generative AI, fueled in large part by the hype surrounding ChatGPT. It and other language models are certainly very powerful, especially for processing unstructured data or as the basis for chatbots and their interactions with customers. For other applications, however, such as optimizing supply chains, they offer hardly any added value.
Solution: AI methods should be selected to serve specific business demands. In many cases the best solution will not be generative AI but a different discipline, as shown by the following three examples: Causal AI helps understand contexts, such as the reasons for unsatisfactory on-time performance by public transportation or quality problems in production. Foresights analytics is a method that enables companies to lower uncertainty levels and anticipate future trends, for instance by improving their demand forecasts or simulating future scenarios. And advanced optimization is a means of identifying optimal outcomes under prescribed framework conditions, such as determining the best routes for containers at ports and thereby optimizing dispatching processes.
Trend 2 Agents know internals
Situation: Language models such as ChatGPT are currently kept very general and trained simply on information publicly available on the internet. They are not adapted to perform specific tasks in a business environment, and do not focus on data of special relevance to concrete applications.
Solution: By using internal data from their respective companies, individual agents can deliver results of precise relevance to the applications at hand. Companies can integrate their data into their individual agents and thereby acquire considerably more effective support. For example, a large language model used in an automotive development context does not immediately understand the technical language in this field. Only after being trained on specific input it can offer real added value. Starting points here include open-source language models such as Google’s Gemini or Meta’s Llama, which experts then modify for specific applications.
Trend 3 Optimization instead of silos
Situation: Many companies want to use AI to gain relevant information from their existing data. This requires dismantling their data silos. In many cases, however, key questions remain unanswered, such as who is responsible for data access and quality. Without a well-functioning form of data governance, even the best AI has no chance of contributing to company success.
Solution: Companies need to lay the foundations for getting the most out of their data. This includes clearly describing who is responsible for which concrete data products and defining this precisely in target agreements. The positive impact of these “enablers” on company success is difficult to measure. But without them, AI solutions will remain well below their potential. Comprehensive transformation processes of this type cover not only the top business priorities and the corresponding concrete data products, but also the company’s AI skills and the responsibilities for AI within its organization.
Trend 4Solutions for Operations
Situation: A saying in the AI field: “Many companies have more pilots than an airline.” In other words, there is often a proliferation of individual, isolated projects run for proof-of-concept purposes. The projects tend not to be integrated into the company as a whole or anchored in sustainable ways. This typical situation leads to problems: too often it is based on the available data instead of the key business challenges that AI is supposed to tackle. Another common problem consists of not having enough expert personnel to manage AI solutions over the long term (for user support, for instance).
Solution: Instead of launching dozens of pilot projects, companies should concentrate on three projects with the greatest impact on their success. And, given the projects’ importance, companies should clearly commit to pursuing them consistently and achieving successful outcomes. A strong focus is crucial here, as is a technical and organizational framework for using AI solutions productively (MLOps). The requisite technical expertise can be developed internally or harnessed from a partner network as needed. As consultants, we support our clients in identifying the right projects, building an initial AI model, and — together with partners — setting up the right processes.
Trend 5The “super mind” combination
Situation: A huge trove of experience lies dormant in companies, located in the minds of their employees. This treasure is neither formulated nor stored in databases. Because AI models lack access to this valuable expert experience, they often do not live up to their potential.
Solution: The key to success lies in combining human expertise with AI models. This yields “super minds.” For example, an AI model might identify a correlation in the course of analyzing data. However, the correlation might actually be incidental, not a relation between cause and effect. Thanks to their experience, human experts can recognize this and thereby help improve the AI model. In many cases, AI models will not be making decisions on their own but rather supporting human decision-making processes. As such, another crucial task for companies is to determine in which areas the “super minds” should only assist and where they should in fact make the decisions. This particular optimization is twofold — of both the AI model as such and its use in everyday company operations.
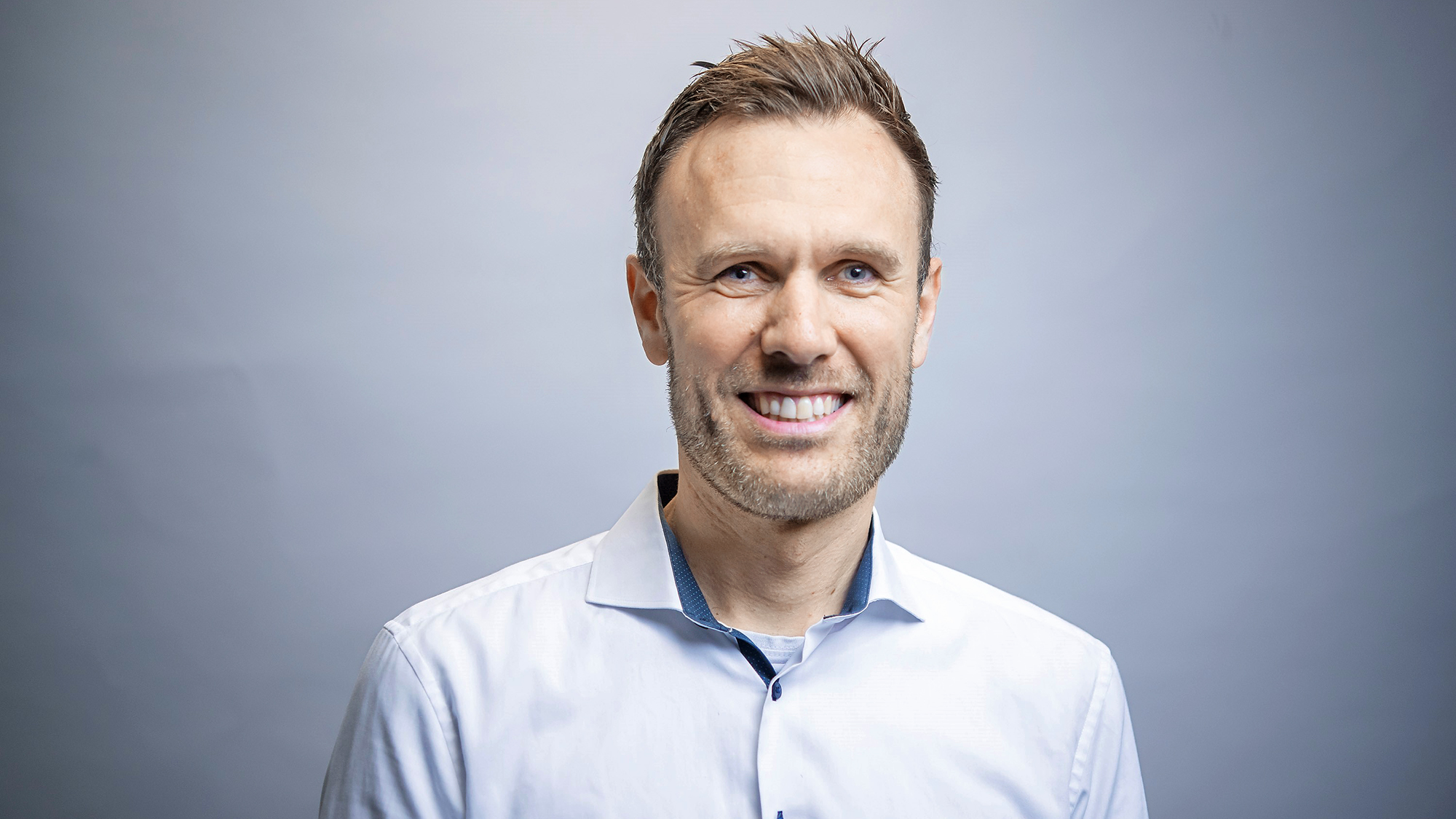