ChatGPT: Promise and Peril
ChatGPT is coming to workplaces. But who commands sufficient knowledge of this language model’s functions, abilities, applications, and risks to make good decisions on whether and how to use it in professional settings?
09/2023
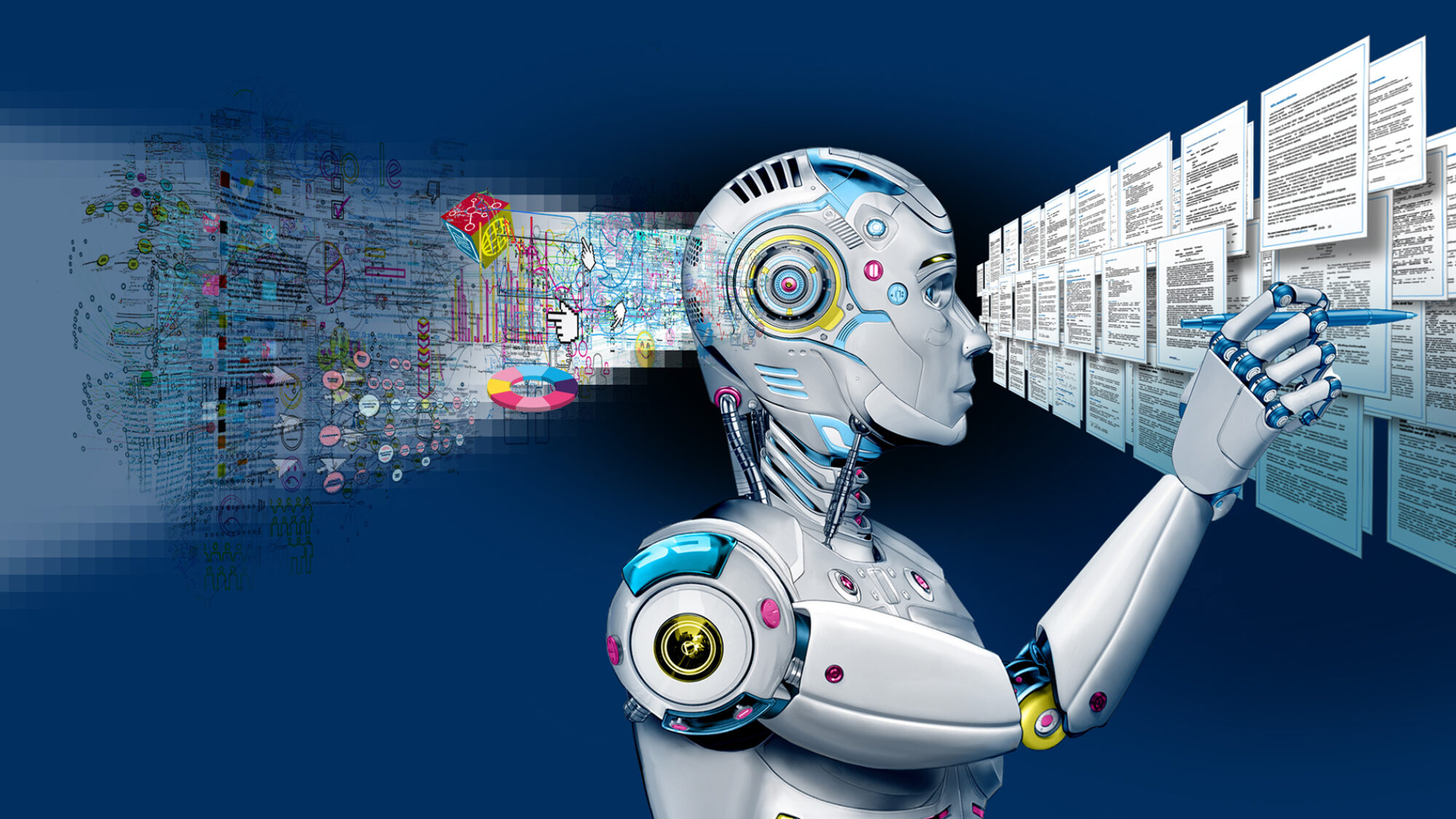
Many large companies are looking to introduce ChatGPT for purposes such as doing research, brainstorming, or generating texts. Some are already using the language-based model, including in customized form. They are hoping artificial intelligence (AI) can reduce employee workloads and increase productivity. But the challenges associated with improving business processes and training personnel in the professional use of their new digital co-worker are sometimes underestimated.
The first step, as everyone would agree, is to acquire a thorough understanding of the chatbot’s basic principles, abilities, and limits. Here, an interdisciplinary team of data analytics and AI experts from the Porsche Consulting management consultancy answers 11 burning questions about ChatGPT and offers solutions based on experience with clients.
Expert answers from Dana, Fabian, and Kevin
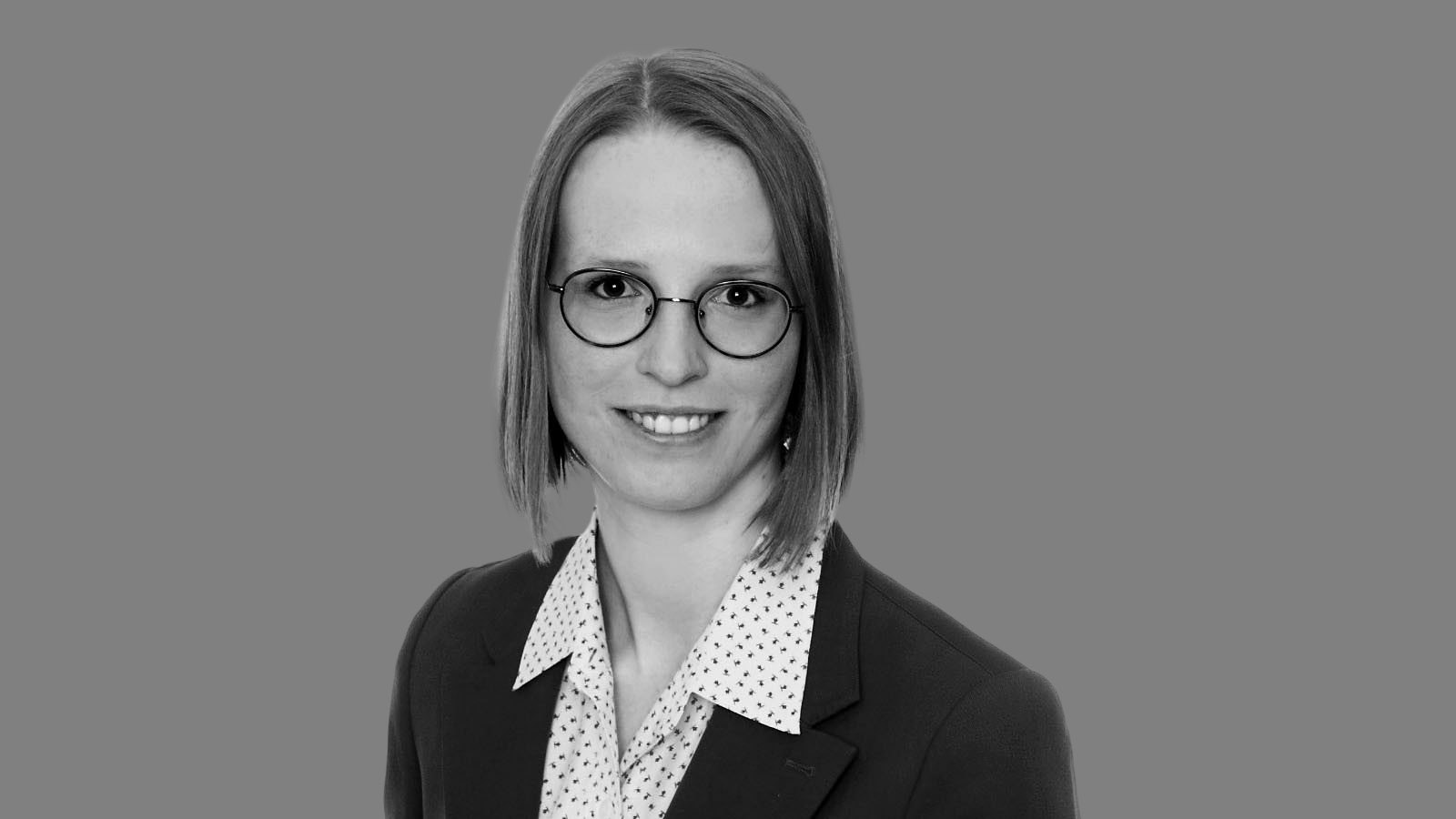
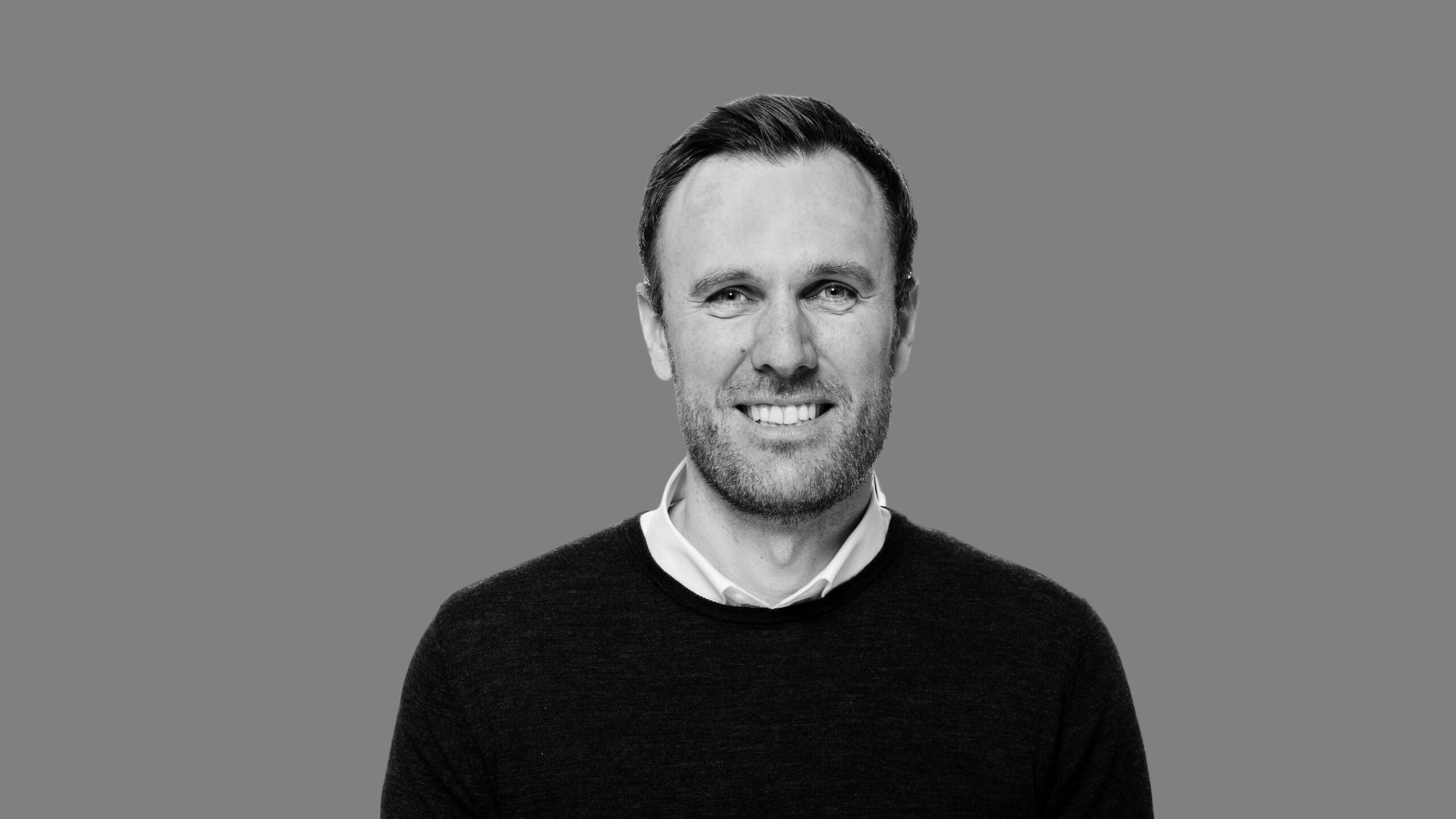
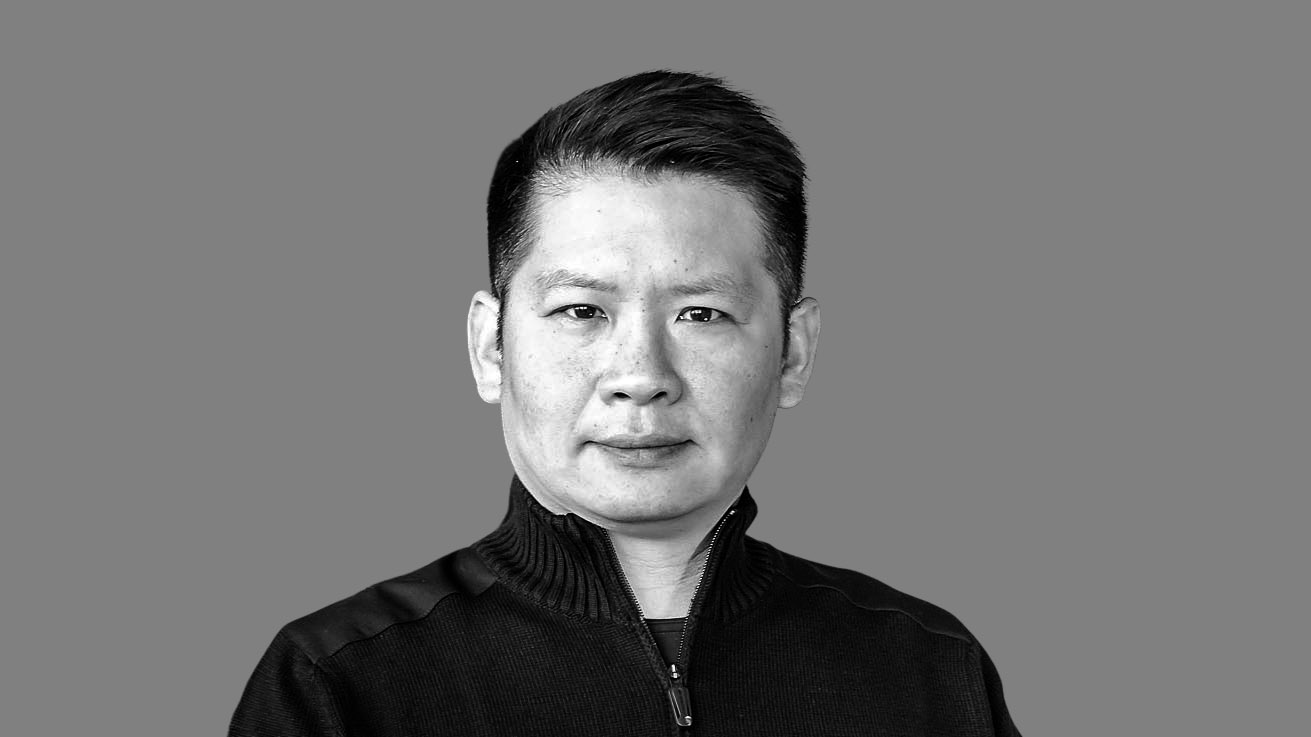
1. What would be the best way of describing ChatGPT?
Dana Ruiter: “ChatGPT is what’s known as a chatbot — basically a language-based model that uses artificial intelligence to simulate language as used by people. ChatGPT has learned how to answer questions in ways that closely resemble human responses. Its answers already seem very natural. But we shouldn’t deceive ourselves: it is a language model, not a knowledge model. And please note that providing factually correct information is not the aim of this model. That being said, large-scale language models do display astonishing abilities to abstract. They can handle a wide array of tasks without having been trained to do so by developers. These large models have made quite a splash not only in AI research circles, but also in the business world.”
2. How does ChatGPT “know” so much? And who “feeds” it?
Dana Ruiter: “There’s a pre-training period, in which the system uses enormous amounts of data from the internet to learn how human language is structured. Then there’s a period of fine-tuning when it learns how to perform certain tasks. ChatGPT’s developers showed it a vast number of question-answer pairs, from which it learned how to simulate answers to questions. The final learning period continues to this day. Human feedback tells the system which answers it generated were useful and which weren’t. That happens every time someone enters a question in ChatGPT and then rates the answer as good or bad. User input is enabling the system to formulate its answers in ever more suitable ways.”
3. Is the system clever enough to generate real “new content,” or is it simply recombining text passages that already exist?
Dana Ruiter: “Large language models have a special quality: The more complex they become, meaning the more training data and neurons they acquire, the more independent abilities they are able to display. They can suddenly do amazing things they haven’t been explicitly trained for. For example, I could ask ChatGPT to translate an informal German email into Shakespearean English. And it can actually do that, although it surely didn’t see that question during its training period. Language models can do this because of the different levels of abstraction they learn. Similar to a brain, actually. The lowest level has very basic information like the meanings of words, for instance. On intermediate levels the words are placed into context, and the ultimate level consists of abstraction. If you ask the system to translate a casual German email into Shakespearean English, it’s already familiar on the abstract level with the concepts of German, Shakespearean English, and translation, so it knows how to formulate the text. This is quite impressive, from a scientific standpoint as well, and was hardly considered possible just several months ago.
But here, too, caution is the name of the game. If the system doesn’t completely understand a term, or if a term inadvertently sends it off in the wrong direction during a task, it can produce incorrect results. These are known in the field as ‘hallucinations.’ They make it hard to apply generative AI models on a stable basis.”
4. Which fields can benefit especially rapidly from ChatGPT? Can the media — whether online, print, or TV — make use of automated news reports? What about contracts for lawyers, medical reports for doctors, or marketing and sales materials for agencies?
Dana Ruiter: “Many fields will benefit greatly from generative AI. There’s a lot of potential especially in areas with high personnel costs such as healthcare and law, and also in administration. The important thing here is to automate repetitive tasks and to let experts concentrate on matters that require their direct attention and knowledge. Let’s take medical exams, for example: doctors can use speech recognition systems to fill out files in advance, which gives them more time to actually talk with their patients. It’s essential, of course, that experts verify everything at the end and decide what actions should then be taken.
The legal profession can also benefit from generative AI. The models can already collect information relevant to a specific question from masses of legal documents, and summarize it as well. Freed from this type of routine work, lawyers can apply their analytical skills to more complex issues.
For the media the problem with generative AI is that it’s not currently connected with world events and is therefore not in a position to automatically produce news reports that are truly innovative and factually accurate. That of course doesn’t stop criminal interests from using AI to generate false news and flood social media with it.”
5. ChatGPT seems to be sparing students a lot of routine work like doing research, compiling sources, or even formulating entire papers. Will digital assistants mean they don’t have to spend time in libraries? And how will this change the face of research and science?
Dana Ruiter: “It’s crucial to keep in mind that ChatGPT is a language model, not a knowledge model. Scientific work consists of observing the world and its properties, and evaluating the knowledge thereby acquired in light of the previous canon of knowledge. ChatGPT can do neither of these things: it can’t observe the world, nor can it reproduce the existing canon of knowledge in factually accurate form. What it’s very good at, however, is simulating the style of a scientific paper. This can be helpful in the formulation stage of a paper once the research results are in and the literature search has been done. But it does not replace the scientific work per se.”
6. ChatGPT users can select different styles and also the degree of in-depth treatment. And if the output doesn’t meet their expectations, they can tell ChatGPT to improve or refine it. What does this tell us about the results — how malleable and how arbitrary are they?
Dana Ruiter: “Results can be manipulated with the help of what’s called prompt engineering. Once again, the problem lies in the system’s lack of transparency and the output’s lack of robustness. The system isn’t transparent because it’s unclear which terms in the prompts have which effects on the sentences generated. It’s pure trial and error. And the lack of transparency means these models are not robust: a prompt that produces the desired result one time can, with slightly modified input, lead to undesirable results otherwise. That makes it especially hard to integrate generative AI models into production systems. After all, no one wants the system to start spouting hate speech or other undesired content due to a trigger in the user input.”
7. In which fields will ChatGPT be used first and most effectively?
Kevin Lin: “ChatGPT is often used as a search machine right now, but I think its main application will be processing documents in automated form. One example would be classifying complaints in order to connect customers with the right advisors or company representatives. Another would be identifying and clearly summarizing relevant administrative information, and yet another would be improving individual writing styles. All these skills are relevant to many different sectors and can easily generate substantial added value. Solutions based on generative AI will be particularly appealing to fields with high personnel costs or a shortage of workers, such as healthcare, the legal profession, or administrative areas.”
8. Which qualifications and jobs will ChatGPT change or even render obsolete?
Kevin Lin: “The working world will change. The good thing here is that specialized work will still need to be done by qualified individuals. ChatGPT cannot replace how a physician interacts with patients or how a lawyer formulates an airtight contract. The ability to integrate specialized expertise with knowledge about the world in general, and to link it with situational and socially aware actions, will remain the domain of well-trained human specialists for quite a while. Repetitive tasks that consistently fit a certain pattern, however, will be replaced to an increasing extent. For services like processing complaints or performing administrative tasks, ChatGPT will offer considerable leverage in terms of increasing efficiency and requiring fewer albeit highly qualified personnel.
Generative AI will eliminate some tasks in the future, that’s clear. Employees will therefore need not only the specialized skills they already command, but also ever greater familiarity with both the abilities and limits of these generative models. There’s a big need here for further training under expert instructors and leaders, right at companies. That’s the only way to ensure that generative AI can be applied in advantageous and dependable ways.”
9. What is ChatGPT not capable of?
Dana Ruiter: “Lots of people confuse ChatGPT with some type of search engine. But that’s not what it is. ChatGPT has only learned to simulate answers to questions. The goals of its training do not include ensuring that these answers are factually accurate. Nor can ChatGPT ensure that the sentences it generates always meet the expectations of the people who entered the prompts. Here’s an example: Let’s say I use ChatGPT to create a tool that helps clients formulate emails in a more professional style. I test my tool, and it seems to work well because the email messages it reformulates sound competent and polite. However, when I hand over the tool to real users who enter all kinds of information, they complain that it neglects parts of their original texts or invents new content. In other words, there’s no guarantee that the system will always act as anticipated. This is why highly sensitive production systems often stick with rule-based processes, which provide certain guardrails for clean and correct output. In the future we’ll be seeing more hybrid systems that combine generative AI with rule-based models in order to make the results more robust.”
10. What alternatives are there to ChatGPT? Are competitors on the horizon?
Fabian Schmidt: “ChatGPT from OpenAI is currently just a chatbot based on a language model. This type of technology is not unique. And there are plenty of competitors. In addition to closed-source models such as Bard from big tech companies like Google, there are also specialized regional solutions from start-ups. In 2022, the Porsche Consulting management consultancy advised Aleph Alpha, an AI start-up from the southwestern German city of Heidelberg, in connection with the German Entrepreneur Award (Deutscher Gründerpreis). Luminous, the language model from Aleph Alpha, is especially relevant for the German market and has a European focus. Its models are hosted in Germany and meet the high European data protection standards. However, there are two major problems with many closed-source solutions. Their models are often not easily adaptable, and data and model sovereignty are frequently in external hands. It’s especially dubious if they’re outside Europe and there’s no way of controlling how the data are processed. That’s why it’s often a good idea for companies to develop their own solutions using open-source models. Some solutions already exist for large-scale language models, such as LLaMA (Meta) and BLOOM (Hugging Face). They’re hosted locally and can be adapted at will, which in turn allows full control of the model and the data. If smaller language models are sufficient — for more basic tasks such as classifying customer complaints or spotting trends on social media — then there’s an entire range of open-source language models such as BERT, XLM, and CamemBERT. Portals like Hugging Face provide free access to these models, along with support functions to facilitate their adaptation to specific use cases.”
11. How can I introduce generative AI solutions at my company?
Fabian Schmidt: “As consultants, the first thing we do with our clients is see whether there’s already a concrete problem that AI should solve. Or whether the goal is to use generative AI to discover new and innovative AI solutions. In the latter case it helps to have a workshop with specialists or to use Porsche Consulting’s InnoLab in Berlin. As soon as we’re clear on the concrete use cases, the decisional processes for how to implement them can begin.
One question that needs to be answered right away is whether it even makes sense to automate a particular task with generative AI. If the answer is yes and an AI solution will save money and time, the next step is to see whether there’s a good ‘off-the-shelf’ solution already on the market. It’s important to note that these solutions don’t have to come from big players like Microsoft or Google. Their solutions tend to be based on very broad usability, which might not be right for a given use case. Smaller companies and established start-ups frequently provide very good solutions for specific use cases and sectors. To gain a good overview here, we recommend doing market analyses that focus on factors like data protection, technical sophistication, and scalability.
If a company cannot find a ready-made solution, however, it needs to develop its own. At Porsche Consulting we work with our clients to clarify the data landscape. Our team’s experts then anonymize and process the data. Only then do we apply the actual model to the use case and refine it together with the client in multiple feedback rounds. For company-specific developments, we place a premium on data and model control, because no one wants their data to fall into external hands. Open-source models that are locally hosted and easily adaptable often offer the surest road to solid company-specific developments.”
Direct contact to Porsche Consulting’s Artificial Intelligence and Data Analytics division: ai@porsche-consulting.com